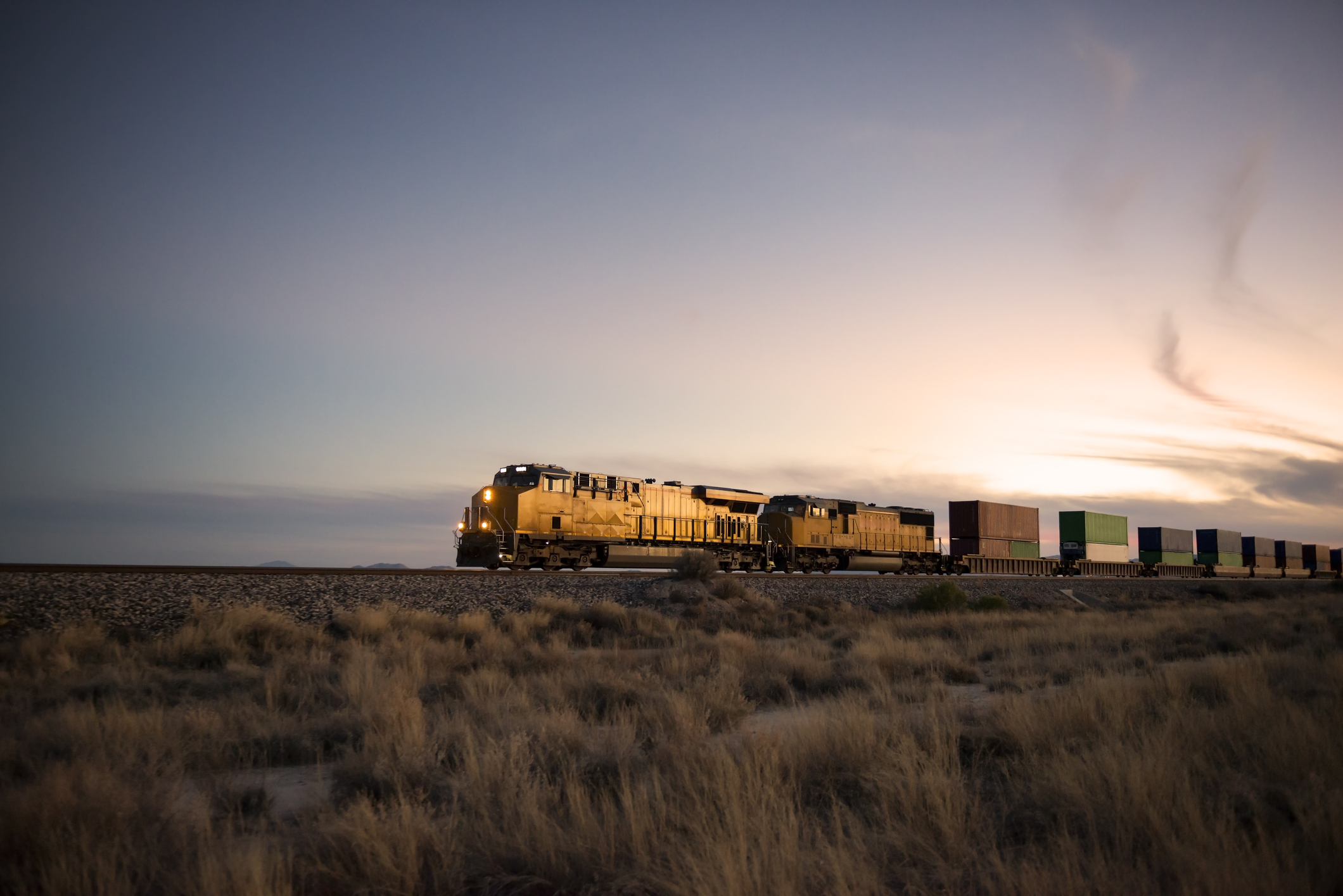
The RailAI® autonomous inspection system delivers highly accurate rail infrastructure data, providing insight into current asset health. AssetAITM models future asset health providing predictive maintenance options, allowing near-term and out-year maintenance plans to be evaluated for minimum risk at lowest cost.
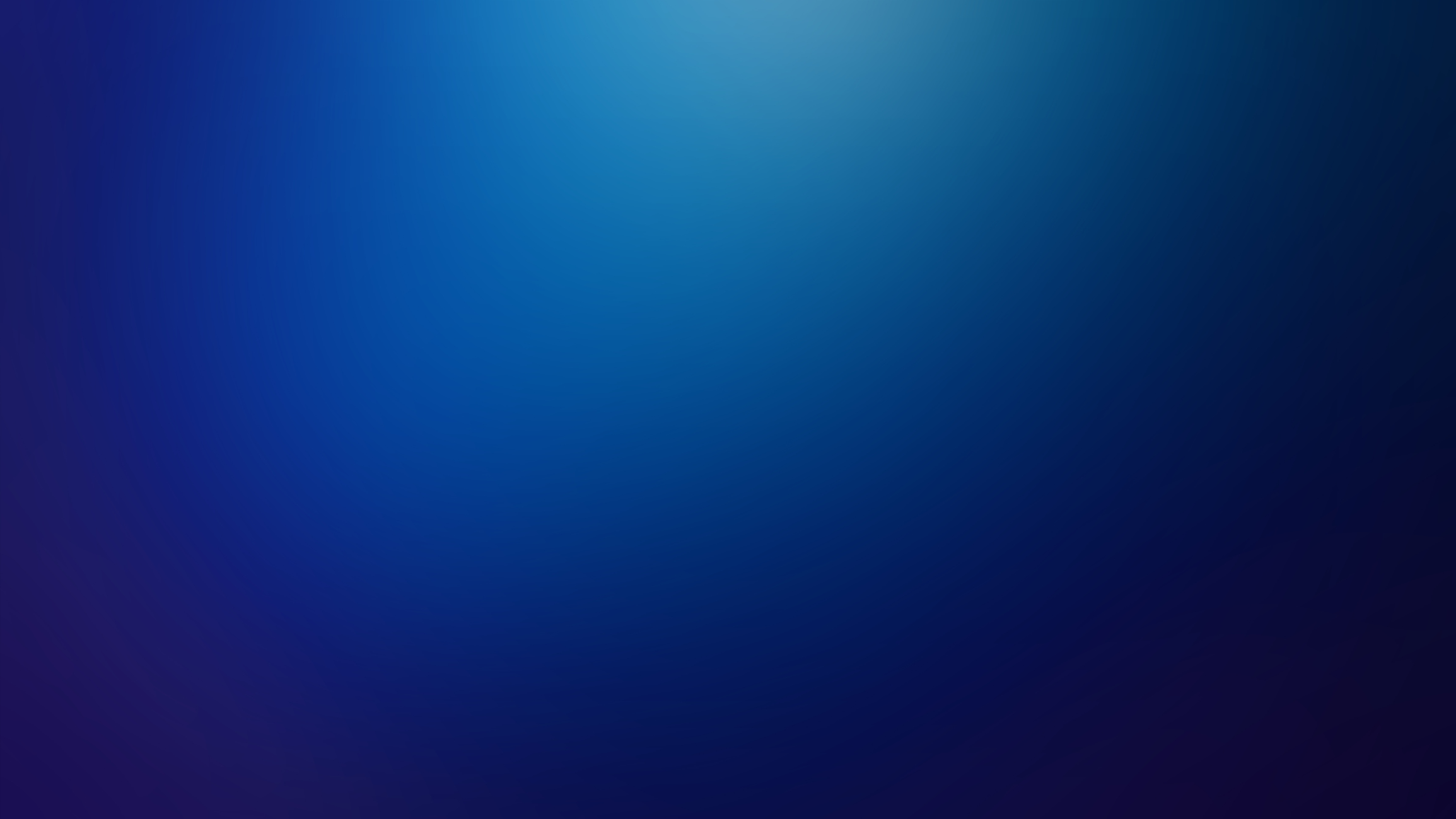
At a Glance
Tie grading and predictive analysis
Rail wear and predictive analysis
Ballast profile and requirement analysis
Substructure modeling
AssetAI in post processing uses RailAI tie grading, rail profile wear, and ballast/subgrade condition data, to provide a modeling and decision aid tool enabling degradation prediction and evaluation of corrective action options.
This allows visualization of degradation over time and risk assessment for impact on track safety standards. The tie grading drill down image illustrates the ability to review data down to the individual tie. Forecasting to year two in the predictive tie condition image shows impact on track safety and the increased probability segments will require slow order if no action is taken as ties degrade.
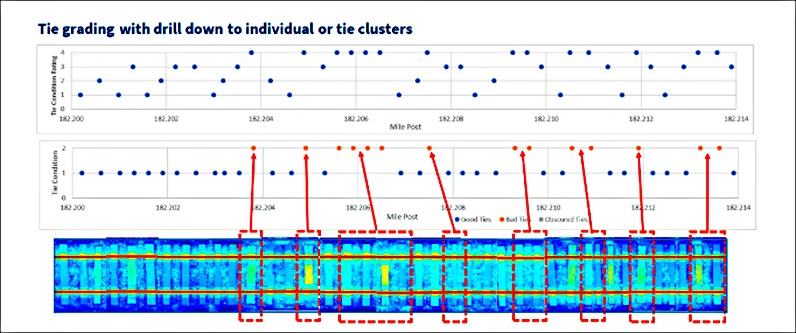
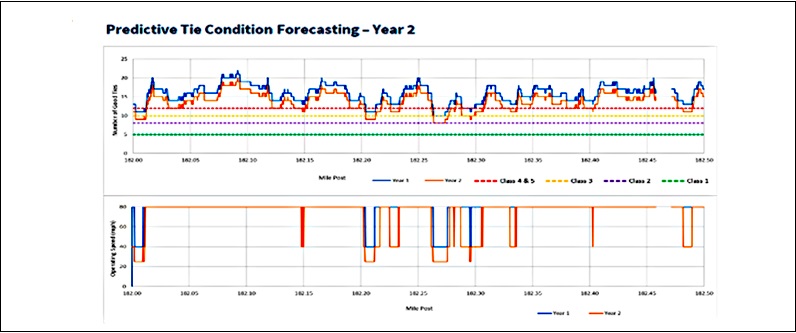
Using the AssetAI predictive health model, clients can model maintenance plans to determine, at an acceptable risk level, what level of annual maintenance will achieve optimum results at the best lifecycle cost.
For example, modeling a full capital tie team replacement in year 1—versus spotting ties for 2 years—then using a capital tie program in year 3, can be compared for cost and risk. Run-over-run data can be used to update the model, improving accuracy of the predictive analytics with each new data set.
Rail profile wear data can provide input for similar rail replacement models, comparing a worn profile to as new in the RailWEB system inventory.
Because the condition of substructure (ballast, sub-ballast, and subgrade) is usually unknown, taking measurements over time is necessary to assess any change. The change, however, can be modeled with adequate data.